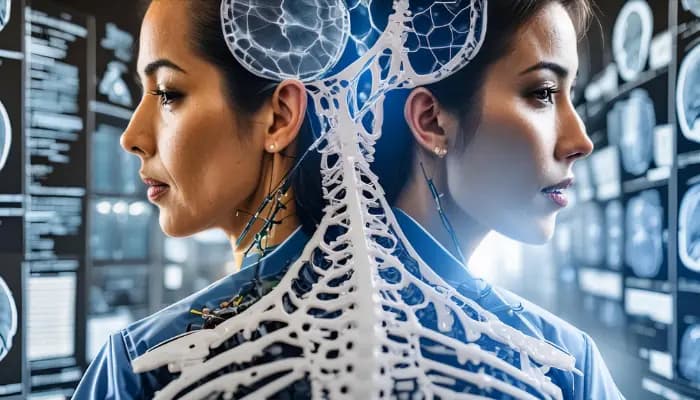
Medical Image Segmentation With UNET
Have you ever thought about how doctors are so precise in diagnosing any conditions based on medical images? Quite simply, it's not alchemy. They rely on sophisticated devices such as U-Net. Which is a deep learning architecture designed for medical image segmentation. It's as if shoving powers in doctors' hands to make them speedy and accurate treatment. And it's simply awesome!
Here in this project, we explore the workings of U-Net and employ it in MRI, CT, and X-ray images. Enjoy the trip through data, coding, and highly advanced medical technology that is greatly helping people.
Project Overview
This is an interesting project that we have taken on as a challenge within the medical field. The task that we seek to address is Medical image segmentation. The task includes accurately marking objects like tumors and organs in the images obtained with MRI, CT, and X−ray using the U-Net model.
U-Net architecture is well-suited for the specific task at hand due to the two-part architecture, It allows images to be segmented at pixel level while maintaining the resolution of the images by capturing all the details. This project shows how to work with medical images, train the U-Net model, and run on the datasets.
Here’s what we'll cover:
- Different image preprocessing techniques
- U-Net model structure and function
- Model training and testing
- The challenges we faced and how to solve them.
Prerequisites
Before embarking on this project, ensure that you possess the following foundational components:
- An understanding of Python programming and usage of Google Colab
- Basic knowledge about deep learning and medical images.
- Comfortable using frameworks like Tensorflow, Keras, Numpy, OpenCV, and Matplotlib to handle data and build models and visualize data and performance of models
- Familiarity with Semantic Segmentation and its role in areas like medical imaging and diagnosis.
- Comfortable with evaluation metrics specifically Mean Intersection over Union (IoU) metrics.
- Availability of jupyter notebook/google colab for the task at hand.
Approach
In this project, we take a detailed step-by-step approach to medical image segmentation using the U-Net model. First of all, the images are loaded and preprocessed for them to be fit for model training.
Then, we design a custom data generator. After that, we can use large datasets without challenges. Then we use flipping and rotation augmentations for further enhancement of the training effort. Next, we build the U-Net architecture. It functions with encodes that downscale the image content and decodes that restore every pixel of the content.
For training the model we use keras. Then we save only the best model callbacks and modification of the learning rate. As training occurs, metrics such as accuracy, mean and standard deviation of IoU are observed to evaluate the model. After training, the U-Net is used to predict segmentation masks. The images then are put into the original images to see how well the model localizes certain areas of interest in the medical scans. At last, the Mean Intersection over Union (IoU) is computed to assess the performance of the predictions for the various classes.
Workflow and Methodology
The overall workflow of this project includes
- Data Collection: In this project, we collect publicly available data containing images and masks.
- Data Preprocessing: Next we process data. Resize, and convert the images to the appropriate color space (HSV, RGB, or grayscale). Then normalize the image to improve model performance
- Model Design: U-Net architecture is designed to perform image segmentation. The encoder is responsible for capturing features, while the decoder works to reconstruct the image at a pixel level.
- Training: Training the U-Net model using the prepared training dataset. The model is evaluated with a validation set to fine-tune values and prevent overfitting.
- Evaluation: We test with the unseen dataset to assess its ability to accurately detect diseases. IoU is used for performance evaluation.
- Visualization: Overlay the predicted segmentation masks onto the original medical images to facilitate easier interpretation of the results.
The methodology involves
- Data Preprocessing: First, images and their corresponding masks are resized to the appropriate input sizes to U-Net architecture. Then pixel values are scaled to the standardized range of 0-1 for the purpose of uniformity.
- Model Architecture: Implemented the U-Net architecture that is most appropriate for this task. Because it preserves the spatial resolution of the input which is good in detail segmentation.
- Metrics: Applied the Mean IoU metric to evaluate the model to make sure that each of the regions in the medical images was correctly segmented.
- Visualization: Showed the results of segmentation by placing the predicted mask on top of the origin image.
Data Collection
First of all, it is necessary to gather a set of RGB images. More so, some preprocessing stages like image resizing can also improve the performance of a model.
Data Preparation
- Resizing: Every image and mask is resized into 128x128 dimensions.
- Normalization: Images are normalized by dividing pixel values by 255 so that they are scaled to a range between 0 and 1.
- Color Conversion: Depending on the dataset, images are converted to different color spaces like HSV, RGB, or grayscale for optimal performance.
- Mask Encoding: In order to assign classes to the encoded masks performed on the RGB image, mapping of pixel values to respective encoded classes is devised.
Data Preparation Workflow
- The images and masks are imported from the dataset.
- Images and masks are rescaled to a suitable size.
- The pixel values are adjusted to a target range.
- The segmentation mask labels are transformed into integers.
- The pre-processed images and masks are then passed to a custom data generator to facilitate training efficiently.
Code Explanation
STEP 1:
Connecting Google Drive
You can mount your Google Drive in a Google Colab notebook. This makes it easy to view files saved in Google Drive. In Colab, you can change and analyze data. You can also train models.
# Mount Google Drive
from google.colab import drive
drive.mount('/content/drive')
Install Necessary libraries
Install libraries like TensorFlow, Keras, and utils. For numerical operations, image processing, machine learning, and visualization.
!pip install keras
!pip install utils
!pip install tensorflow
Import Necessary libraries
Import necessary libraries like numpy, tensorflow, matplotlib etc. These libraries will help with computational processes. Also, it will help to build and train models. After that, we can visualize results through these libraries.
import numpy as np
from tensorflow.keras.utils import Sequence
import cv2
import tensorflow as tf
import pickle
from tensorflow.keras.preprocessing.image import ImageDataGenerator
import sklearn
from sklearn.cluster import KMeans
from tensorflow.keras.layers import *
from tensorflow.keras import models
from tensorflow.keras.callbacks import *
import glob2
from sklearn.utils import shuffle
import matplotlib.pyplot as plt
from tensorflow.keras.metrics import MeanIoU
STEP 4:
Defines utility functions cvtColor and func.
Here is the code of two utility functions. If the values of the pixels are less than 255, the first function ('cvtColor') sets the values to 0. Applying the 'cvtColor' function to every pixel in an image is what the second function ('func') does.
# Define a function to convert color space to RGB
def cvtColor(x):
x[x < 255] = 0
return x
# Define a function to apply cvtColor to each pixel in the image
def func(img):
d = list(map(lambda x: cvtColor(x), img.reshape(-1,3)))
return np.array(d).reshape(*img.shape[:-1], 3)
DataGenerator Class for Batch Processing and Preprocessing
The DataGenerator class generates data batches for model training, with its constructor initializing arguments like data filenames, input and batch sizes, shuffle options, color mode, encoding dictionary, and optional processing functions. The processing method encodes masks using the provided dictionary. The __len__ method calculates the number of batches per epoch based on dataset and batch size, while the __getitem__ method retrieves a subset of filenames by batch index and uses data_generation to load and preprocess images and masks. The on_epoch_end method updates indices and shuffles them after each epoch. Finally, the data_generation method handles loading and preprocessing images and masks, adjusts sizes, manages color modes (HSV, RGB, grayscale), applies optional processing, and normalizes pixel values for the current batch.
class DataGenerator(Sequence):
def __init__(self, all_filenames, input_size=(128, 128), batch_size=8, shuffle=True, seed=123, encode: dict = None, color_mode='hsv', function=None) -> None:
super(DataGenerator, self).__init__()
# Check if the encoding dictionary is provided
assert encode != None, 'Not empty !'
# Check if the color mode is valid
assert color_mode == 'hsv' or color_mode == 'rgb' or color_mode == 'gray'
# Initialize instance variables
self.all_filenames = all_filenames
self.input_size = input_size
self.batch_size = batch_size
self.shuffle = shuffle
self.color_mode = color_mode
self.encode = encode
self.function = function
# Set random seed for shuffling
np.random.seed(seed)
# Shuffle the data at the start
self.on_epoch_end()
def processing(self, mask):
# Encode mask based on the provided dictionary
d = list(map(lambda x: self.encode[tuple(x)], mask.reshape(-1, 3)))
return np.array(d).reshape(*self.input_size, 1)
def __len__(self):
# Calculate the number of batches per epoch
return int(np.floor(len(self.all_filenames) / self.batch_size))
def __getitem__(self, index):
# Generate one batch of data
indexes = self.indexes[index * self.batch_size : (index + 1) * self.batch_size]
all_filenames_temp = [self.all_filenames[k] for k in indexes]
X, Y = self.__data_generation(all_filenames_temp)
return X, Y
def on_epoch_end(self):
# Update indexes after each epoch
self.indexes = np.arange(len(self.all_filenames))
if self.shuffle == True:
np.random.shuffle(self.indexes)
def __data_generation(self, all_filenames_temp):
# Generates data containing batch_size samples
# Initialize arrays for images and masks
batch = len(all_filenames_temp)
if self.color_mode == 'gray':
X = np.empty(shape=(batch, *self.input_size, 1))
else:
X = np.empty(shape=(batch, *self.input_size, 3))
Y = np.empty(shape=(batch, *self.input_size, 1))
# Iterate over the filenames in the current batch
for i, (fn, label_fn) in enumerate(all_filenames_temp):
# Load and preprocess image
img = cv2.imread(fn)
if self.color_mode == 'hsv':
img = cv2.cvtColor(img, cv2.COLOR_BGR2HSV)
elif self.color_mode == 'rgb':
img = cv2.cvtColor(img, cv2.COLOR_BGR2RGB)
elif self.color_mode == 'gray':
img = cv2.cvtColor(img, cv2.COLOR_BGR2GRAY)
img = tf.expand_dims(img, axis=2)
img = tf.image.resize(img, self.input_size, method='nearest')
img = tf.cast(img, tf.float32)
img /= 255.
# Load and preprocess mask
mask = cv2.imread(label_fn, 0)
mask = cv2.cvtColor(mask, cv2.COLOR_BGR2RGB)
mask = tf.image.resize(mask, self.input_size, method='nearest')
mask = np.array(mask)
if self.function:
mask = self.function(mask)
mask = self.processing(mask)
mask = tf.cast(mask, tf.float32)
# Assign images and masks to the arrays
X[i,] = img
Y[i,] = mask
return X, Y
Converts pixel-wise labels and saves the mapping in a pickle file
The 'encode_label' function transforms a mask into a 2D array of pixel values, creating unique labels. Constructs an encoder dictionary, and saves the dictionary to a pickle file, preserving the mapping for later use.