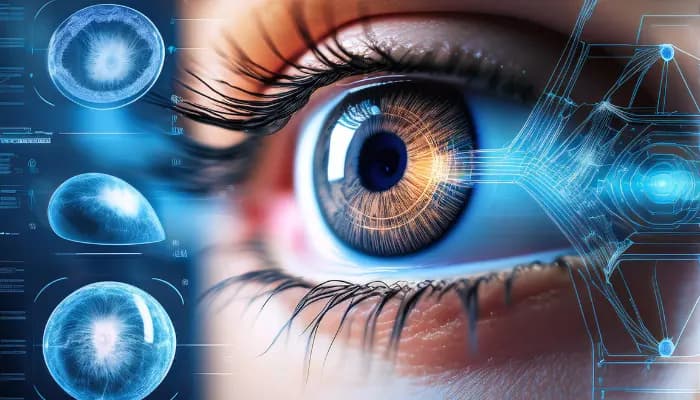
Automatic Eye Cataract Detection Using YOLOv8
Cataracts are a leading cause of vision impairment worldwide, affecting millions of people every year. Early detection and timely intervention can significantly improve the quality of life for those at risk. However, manual detection methods can be time-consuming and prone to human error. To address this challenge, we present the Automatic Eye Cataract Detection system. This project leverages advanced computer vision techniques and the YOLOv8 model to automate the detection of cataracts from eye images, providing an efficient, accurate, and scalable solution. By integrating this technology into healthcare, we can facilitate early diagnosis and help reduce the burden of cataract-related blindness.
Project Overview:
In this project, we will learn how to build Automatic Eye Cataract Detection using YOLOv8 with a high-accuracy, AI-powered solution for efficient automatic cataract detection. Improve eye care diagnostics with our scalable and user-friendly system. The goal is to develop an AI-powered tool that can quickly and accurately identify cataracts in eye images and videos. Detecting early cataracts is crucial for eye health, and this tool aims to enhance the speed and reliability of the health sector.
Prerequisites
Before we get started, here's what we will need:
-
Sound knowledge of Python and Machine Learning concepts.
-
Knowledge and familiarity with Yolo, which is a widely used object detection model.
-
Make sure you install roboflow, ultralytics, and gradio to manage datasets, train models, and create a simple web interface.
-
Good understanding in libraries like pandas, numpy for processing data, matplotlib for visualization, and a basic understanding of evaluation metrics. It includes mAP (mean Average Precision), F1-score, and precision-recall curves. It will help you to measure the model's performance.
Approach
In this project to detect a cataract, we will be employing a pre-trained version of the YoloV8 Nano model owing to its lightweight frame as well as its quick processing speeds. To begin with, we shall compile a dataset that includes both healthy and diseased cataract images, followed by training and validation sets' preparation and resizing of the images. Soon after training the model, we'll be keeping track of the model performance with some metrics e.g. mAP (Mean Average Precision). After successful training of neural networks, we will evaluate their performance with mAP and precision. For the convenience of the users, we will create an online interface on Gradio to be available for real time - cataract detection which will then be tested and made available for healthcare purposes.
Workflow and Methodology
Here's the workflow we'll follow:
- Data Collection: We start by collecting a dataset from Roboflow. The dataset consists of both healthy and cataract-affected.
- Data Preparation: After collecting the dataset, we prepare it for training. For YoloV8, images were resized in 64x64 pixels. Because this is the ideal size for the YoloV8 model.
- Model Training: Once the dataset is complete, we train the model on this dataset with YOLOv8, going through 100 training epochs to improve its ability to detect cataracts.
- Model Validation: After training, we validate the model to assess its accuracy in distinguishing between cataracts and normal eyes. We calculate key metrics such as mAP and precision to evaluate the model's performance.
- Deployment: Finally, we use Gradio to set up a user-friendly interface where individuals can upload their images or videos and receive real-time predictions.
Data Collection
We're gathering our dataset from Roboflow, which simplifies the process of downloading a ready-to-use dataset in YOLO format. This dataset contains both normal eye images and those affected by cataracts, allowing the model to learn how to distinguish between the two.
Data Preparation
Before we can input the data into the model, we need to get it ready. We resize all images to 640x640 pixels, which is the preferred size for YOLO.
Data Preparation workflow
- Downloading the dataset for cataract detection from Roboflow, a platform that offers high-quality datasets for machine learning.
- A connection to Roboflow is made via a unique API key. The API key provides access to the dataset repository and ensures secure data retrieval.
- The "Kataract Object Detection." The project is accessed. The project includes a labeled dataset. It was developed for cataract object detection.
- The third version of the YOLOv8 configured database has been obtained. It is pre-configured and tuned to work with the YOLOv8 architecture.
Code Explanation
STEP 1:
Mount Google Drive
Mount your Google Drive to access and save datasets, models, and other resources.
from google.colab import drive
drive.mount('/content/drive')
Installing Necessary Packages
Installs the necessary python packages. Roboflow for managing and downloading dataset, ultralytics provides YOLOv8 for object detection and gradio to create a user-friendly interface.
!pip install roboflow
!pip install ultralytics
!pip install gradio ultralytics
Downloading the Dataset from Roboflow
Here, the dataset is downloaded from Roboflow and an API key is required to authenticate with the platform. The "newworkspace-t5oqu" refers to the workspace ID where the project resides.
Additionally, Roboflow allows users to maintain multiple versions of a dataset. In this case, version 3 of the "kataract-object-detection" project is accessed. Afterward, the dataset is saved locally and is now ready to be used for training the YOLOv8 model.
from roboflow import Roboflow
rf = Roboflow(api_key="PYIrYvf8WFPOSaItVRYl")
project = rf.workspace("newworkspace-t5oqu").project("kataract-object-detection")
version = project.version(3)
dataset = version.download("yolov8")
STEP 2:
Imports necessary library
The libraries such as PIL, opencv, matplotlib, and numpy are imported to handle image input/output, image processing, visualization, and gradio for creating web interfaces.