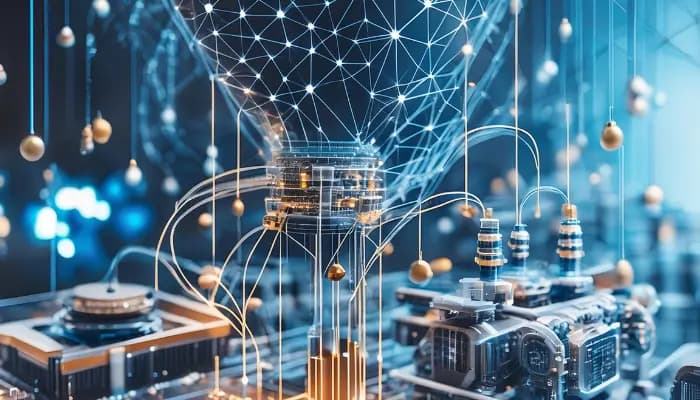
Image Generation Model Fine Tuning With Diffusers Models
Imagine art graphics being created with a few clicks! That is what this project is about. We are exploring image generation, the art of Diffusers, and Stable Diffusion in order to transform your imagination into something real.
Project Overview
This project also enhances the image generation aspect. We use Diffusers to fine-tune specific pre-trained models so that it generate crisp and high-resolution images at a faster rate. But we don’t stop there. Everything is modified – learning rates and prompts to suit your requirements. The trained model is converted back to the stable diffusion format for easier applications.
You can easily use a Gradio interface where you can input prompts and then you’ll view the images. For instance, imagining a man running a marathon in outer space, or any other is exaggerated, this project does it all!
Buckle up for an experience and adventure as we involve technology in art.
Prerequisites
Before we dive into the code, Here’s what we’ll need:
- Knowledge of Python programming.
- Understanding of deep learning and neural networks.
- Google Colab or GPU selection.
- Awareness of the setting of the GPU in Colab or using a local CUDA device.
- Familiar with Hugging Face and Gradio.
- Having image processing knowledge in terms of resolution and pixel size.
- Controlling CUDA, and GPU ( allocation of memory, monitoring the devices using nvidia-smi).
- Understanding of Diffusers and Stable Diffusion models
Approach
The strategy of this work revolves around improving the image generation process using a systematic fine-tuning approach. In the first steps, a suitable image generation model is fine-tuned using Diffusers. This makes it easier to produce better images by modifying hyperparameters such as the learning rates and batch sizes. The model is further augmented through the use of data augmentation techniques, and the training schedule is designed to be adjustable, depending on the demands. When the model is set up, it is converted to the Stable Diffusion format to make it compatible with widely used diffusion-based frameworks. The project also provides a Gradio interface, which allows to generate images interactively by providing prompts. This makes the process intuitive and easy and also conducive to massive datasets and plenty of scenarios. Such parameters, for instance, loss values and sample images, help to control the process of work and ensure the quality of results during the entire training period.
Workflow and Methodology
The workflow of this project includes several key steps, making it easy to follow:
- Environment Setup: To begin with, the required libraries were installed with the help of pip.
- Datasets Collection and Preparation: The first stage consists of collecting the data set which has a variety of images.
- Model Fine-Tuning: We started with a preloaded model and used a Diffuser model to increase image generation efficiency.
- Training Process: Data augmentation, model optimization, and flexible parameter tweaking were used to gain additional proficiency in the model.
- Conversion: After the training was over we switched the model back to the Stable Diffusion format.
- Interactive UI: In the end, we have developed a Gradio interface for creating new images with bespoke prompts.
Data Collection
In this project, the image collection can be considered a very important phase of the project. You will need to take a relevant number of serial pictures. Make sure that the images show your face such that different views are covered. Since it is ideal to have a minimum of 25 images. After going through the images, keep the balance and discard. This helps provide diversity. It is significantly important in improving the robustness of the model when fine-tuning.
Data Preparation
For this project, you will be the one who will take the images to make the dataset. From different positions, it is important that the image has to be taken. The quality of the images is very important. Hence it is recommended that only the good images be set aside for use in the future. These images will then be worked upon and made ready for the training of the diffuser-based model.
Data Preparation Workflow
- Image Capture: All the images must be taken from different viewpoints. Ensure that multiple pictures are taken from various angles.
- Image Sorting: All the images taken through the camera in positive or negative directions. It must be analyzed and the most perfect images must be chosen.
- Final Dataset: Make sure that at least 25 images, which are to the best capture and in terms of model training. Possible Images are from different angle positions relative to the subject-oriented images. That is retained in the final dataset for modeling purposes.
Explanation All Code
STEP 1:
Gathering GPU information
In this code, we utilize commands to query GPU information using NVIDIA System Management Interface (nvidia-smi). This ensures that the environment is set up correctly for training.
# Command to query GPU information (name, total memory, and free memory) using NVIDIA System Management Interface (nvidia-smi)
# The output is formatted in CSV format with no header
!nvidia-smi --query-gpu=name,memory.total,memory.free --format=csv,noheader
Two Python scripts are downloaded from a GitHub repository. One for training models. And another for converting Diffusers models to the original Stable Diffusion format. Next, it clones the Diffusers library from GitHub and then updates the Triton library for the purpose of further deep-learning improvements.
Moreover, some more packages like `accelerate`, `transformers`, `ftfy`, `bitsandbytes`, `gradio`, and `natsort` are installed, which help in the optimization of training using GPUs, text handling and in developing interfaces respectively.
# Downloading the training script for the DreamBooth project from the Diffusers GitHub repository
!wget -q https://github.com/ShivamShrirao/diffusers/raw/main/examples/dreambooth/train_dreambooth.py
# Downloading the script to convert Diffusers models to the original Stable Diffusion format
!wget -q https://github.com/ShivamShrirao/diffusers/raw/main/scripts/convert_diffusers_to_original_stable_diffusion.py
# Installing the Diffusers library from the GitHub repository
%pip install -qq git+https://github.com/ShivamShrirao/diffusers
# Upgrading the Triton library to the latest pre-release version
%pip install -q -U --pre triton
# Installing additional Python packages required for the project
%pip install -q accelerate transformers ftfy bitsandbytes gradio natsort
STEP 2:
Input The Huggingface Token
This script helps to configure the Hugging Face authentication as it defines a hidden directory if one does not exist; \~/.huggingface. It then translates the token Hugging Face API token, set for use in other parts of authentication. Last of all, the token is stored in the file token under the directory \~/.huggingface, which is necessary for using Hugging Face services, such as downloading or using models.
# Create a directory ~/.huggingface if it doesn't exist
!mkdir -p ~/.huggingface
# Set Hugging Face token for authentication
HUGGINGFACE_TOKEN = "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"
# Write the Hugging Face token to a file named token within the ~/.huggingface directory
!echo -n "{HUGGINGFACE_TOKEN}" > ~/.huggingface/token
This block sets the model name and output directory for saving trained weights, optionally mounting Google Drive for storage and ensuring the output directory exists.
# Boolean flag to indicate whether to save to Google Drive
save_to_gdrive = True
# If save_to_gdrive is True, mount Google Drive to the Colab environment
if save_to_gdrive:
from google.colab import drive
drive.mount('/content/drive')
# Name/Path of the initial model
MODEL_NAME = "stabilityai/stable-diffusion-2"
# Define the directory name to save the model weights
OUTPUT_DIR = "stable_diffusion_weights/aionlinecourse"
# If save_to_gdrive is True, set the output directory path to be within the Google Drive
if save_to_gdrive:
OUTPUT_DIR = "/content/drive/" + OUTPUT_DIR
else:
# Otherwise, set the output directory path to be within the Colab environment
OUTPUT_DIR = "/content/" + OUTPUT_DIR
# Print the path where the weights will be saved
print(f"[*] Weights will be saved at {OUTPUT_DIR}")
# Create the output directory if it doesn't already exist
!mkdir -p $OUTPUT_DIR
A list of dictionaries representing concepts is stored in the concept_list variable. It is specifying prompts and data directories. It creates necessary directories for instance data and writes the concepts list to a JSON file.
# Define a list of dictionaries representing concepts
concepts_list = [
{
"instance_prompt": "art by aionlinecourse",
"class_prompt": "art by a person",
"instance_data_dir": "/content/drive/stable_diffusion_weights/aionlinecourse",
"class_data_dir": "/content/data/artbyperson"
}
]
# Import the json and os modules
import json
import os
# Create directories specified in the instance_data_dir attribute of each concept dictionary
# If the directories already exist, no error will be raised (exist_ok=True)
for c in concepts_list:
os.makedirs(c["instance_data_dir"], exist_ok=True)
# Write the concepts_list to a JSON file named concepts_list.json with indentation for readability
with open("concepts_list.json", "w") as f:
json.dump(concepts_list, f, indent=4)
STEP 3:
Upload the training data
This code uploads instance images from the user's local system to the appropriate instance data directory for every idea listed in the 'concepts_list'. For every loop, it prints a message indicating the uploading process. 'shutil.move()' is used to relocate each file to its assigned directory after uploading.