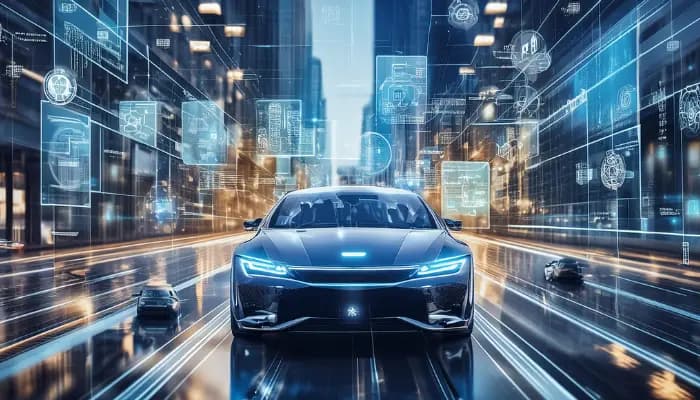
Real-Time License Plate Detection Using YOLOv8 and OCR Model
Ever wondered how those cameras catch license plates so quickly? Well, this project does just that! Using YOLOv8 for real-time license plate detection and OCR models, it recognizes the text in the plates. You'll get to dive into the world of computer vision, where detecting and reading license plates becomes a breeze. Whether you're into AI projects or just curious, this one's going to be a great work!
Project Overview
This project takes license plate detection to the next level with YOLOv8. Yolov8 is a super-fast object detection model. The goal is to detect license plates in images and then extract the text from them using OCR models. It’s perfect for building smart systems, like parking lots or toll booths, that need to recognize vehicles automatically. Whether you're working with images or video streams, it handles both with ease. The project is designed to be fast, accurate, and easy to use. Plus, it’s scalable.
Key Features:
- YOLOv8 is fast and precise. It detects plates instantly in real-world conditions.
- The project doesn’t just detect plates, it reads them! OCR helps extract the plate number quickly.
- With high accuracy, this system minimizes mistakes in license plate recognition, making it dependable.
- Built with simplicity in mind, you can easily integrate it into any system or application.
Prerequisites
Before you dive into this fun project, here are a few things you'll need to have ready:
- Ensure that Python is installed (recommended to use at least the third version).
- For license plate detection, you will need to install YOLOv8 from Ultralytics.
- Don’t forget to integrate an OCR library that will help to read the text of the license plate, for example, Tesseract or EasyOCR.
- The OpenCV library will be associated with image processing, and it will be helpful to display the results in real-time.
- Ensure that you have pip or conda to install the necessary Python packages as the installation process will be easy.
- You don’t have to be a programming expert, although any background in Python programming will assist with reading and tweaking the code.
- Join RoboFlow and sign up for a free account and organize, label, and annotate your data set. RoboFlow helps in simplifying the preparation of the data for YOLOv8.
Approach
Here in this project, we apply YOLOv8 to detect license plates in the images and video stream. The Yolov8 model is trained for license plate detection. This model guarantees a high level of detection and accuracy. Once the license plates are detected, the Optical Character Recognition tools such as Tesseract or EasyOCR extract the text out of those plates. For real-time image processing and visualization, OpenCV is employed to handle the input and display the results. The dataset for training and testing is managed and labeled using RoboFlow. This approach effectively combines YOLOv8 for object detection with OCR for text recognition. This enables fast and accurate detection and reading of license plates, even in challenging conditions.
Flow of Actions
The overall workflow of this project includes:
- Data Collection: We start by collecting a dataset from Roboflow.
- Data Labeling and Preprocessing: Before the detection of the license plate images, they were labeled using the RoboFlow.
- Model Setup: Images went through a pre-trained YOLOv8 model for detecting license plates.
- Detection Phase: This project used the YOLOv8 model to detect and localize license plates on the input images.
- OCR Integration: Tesseract or EasyOCR was used to extract the numbers from the license plate.
- Image Processing: For managing input images and displaying the detection results OpenCV was used.
- Text Output: Text was extracted from the license plate and further preprocessed and exclaimed in visual form.
Data Collection
For this project, the dataset used was obtained from publicly available license plate image datasets from Roboflow. These images were then analyzed using RoboFlow for labeling and making the images fit for training YOLOv8 models. No custom data collection was performed. The project collected the dataset that was primarily developed for license plate detection and recognition challenges.
Data Preparation
The dataset involved in this project comprises vehicles with clear number plates. To label these images, the RoboFlow was used to draw the exact location of the plate. To make the dataset accurate, the images were taken under different lighting conditions, at different angles, and perspectives. After labeling, the data was split again into train and test so as to train the YOLOv8 model for license plate detection and testing the performance of the model. Such a preparation phase contributed to a model capable of identifying license plates in different scenarios in a real-world setting.
Data preparation workflow:
- Dataset Upload: Users uploaded vehicle images showing their number plates to RoboFlow for further processing.
- Annotation: All the images were annotated in RoboFlow by indicating various positions of the number plates for proper training of the intended model.
- Data Augmentation: Increase the dataset with image variations (rotate, crop, brighten, etc.) to improve the reliability of the model.
- Data Splitting: The labeled data was divided into two parts: training data to train the model and test data to evaluate the trained model.
- Exporting Dataset: At last, the dataset was exported into a format that can be used with the YOLOv8 model in preparation for the training of the model.
Step-by-step guide:
STEP 1:
Connect Google Drive
You can mount your Google Drive in a Google Colab notebook with this piece of code. This makes it easy to view files saved in Google Drive. Additionally, in Colab, you can change and analyze data. You can also train models.
from google.colab import drive
drive.mount('/content/drive')
The os.chdir() function sets the current working directory to the specified path. This means that the code will now operate in this folder for any file-related tasks. The os.getcwd() function is used to print the current working directory. This confirms that the script has successfully moved to the specified directory.
import os
# Set the current directory
os.chdir("/content/drive/aionlinecourse/real_time_license_plate_detection_and_using_ocr")
# Verify the change in the current directory
os.getcwd()
STEP 2:
Install Necessary Packages.
This code installs the Ultralytics library. It provides access to the YOLOv8 model for object detection. After installation, it imports the library and runs a system check using ultralytics.checks() to ensure the environment is properly set up and all dependencies are ready for running YOLOv8.
%pip install ultralytics
import ultralytics
ultralytics.checks()
STEP 3:
Load dataset
This code trains the YOLOv8 model on a license plate dataset for 100 epochs using images sized at 640x640 pixels.
!yolo train model=yolov8n.pt data= "/content/drive/aionlinecourse/real_time_license_plate_detection_and_using_ocr/dataset/data.yaml" epochs=100 imgsz=640
STEP 4:
Install the necessary packages.
This code imports libraries required for image processing and object detection, including torch for deep learning, OpenCV for handling images, Ultralytics for using the YOLOv8 model, PIL for image manipulation, torchvision transforms for image transformations, and matplotlib for visualizing results.
import torch
import cv2
import ultralytics
from cv2 import imread
from ultralytics import YOLO
from torchvision import transforms
from PIL import Image
from matplotlib import pyplot as plt
STEP 5:
Choosing an AI Model for Real-Time License Plate Detection
For this project, YOLOv8 was chosen due to its exceptional ability. To perform real-time object detection with this model. YOLO models are known for their speed and accuracy in identifying objects within images. YOLOv8 processes number plate images in a single deep layer. It detects and identifies number plates. After that, OCR extracts the main text from number plates for license plate recognition.
By using YOLOv8, we ensured that the model aligns with the project’s goal. It provides fast, accurate, and detection. Also, it enhances the detection capabilities in self-driving applications.
Load a pretrained YOLOv8n model
This code loads a pre-trained YOLOv8n model from the specified path using Ultralytics.