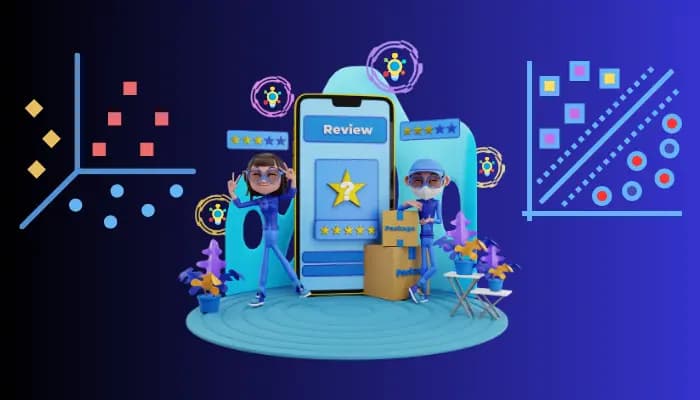
Topic modeling using K-means clustering to group customer reviews
Have you ever thought about the ways one can analyze a review to extract all the misleading or useful information? The present project is about analyzing customer reviews through sentiment analysis, topic modeling, or clustering.
Project Overview
The goal of this project is to study consumer reviews and use them creatively to derive useful insights. Reviews are first processed and cleaned using NLTK and Scikit-learn. Next, these reviews attribute sentiments such as positive, neutral, or negative depending on the rating given using models such as Random Forest and Naive Bayes to mention a few. But wait! Thanks to LDA, we can also do some topic modeling and learn what topics are present but not visible. K-Means is a clustering technique that allows us to analyze and interpret a set of clusters formed by several similar reviews. Last but not least, we make very creative visualizations such as word clouds and sentiment heat maps. What a wonderful way to demonstrate the potential of data!
Prerequisites
Learners must develop some skills before undertaking this project. Here’s what you should ideally know:
- Python version 3.7 or higher installed on your system.
- Understanding of basic knowledge of Python for data analysis and manipulation
- Knowledge of libraries such as NLTK, Gensim, Scikit-learn, Pandas, NumPy, Seaborn, Matplotlib, pyLDAvis, and WordCloud is necessary.
- The dataset consists of customer review data with Rating and Review columns.
- Jupyter Notebook, VScode, or a Python-compatible IDE.
Approach
The structure of the project begins with data preprocessing works, which include cleaning, tokenizing, and lemmatizing of the reviews. Tools such as NLTK are Used in conducting this activity to maintain consistency across the reviews. After that in the process of review analysis machine learning methods like Random Forest, Naive Bayes, and others are used to divide the reviews into positive, neutral, and negative. Then, LDA – an advanced Bayesian technique for topic modeling – is used to analyze customer reviews to identify more themes in the customer feedback. K-Means is also implemented to cluster the reviews to facilitate the identification of the trends and patterns. Adequate infographics such as word clouds, sentiment heat maps, and clustering plots are also provided for a better understanding of the analysis. This disciplined methodology guarantees thorough inquiry of customer reviews.
Workflow and Methodology
Workflow
- Data Collection
- Obtain consumer reviews with specific columns: Rating and Review
- Data Preprocessing
- Edit content materials by deleting, for instance, punctuation marks, numbers, and even stopwords.
- Using NLTK perform text tokenization and lemmatization for text standardization.
- Exploratory Data Analysis (EDA)
- The distribution of ratings and the lengths of reviews will be examined.
- The frequency of certain words and the most popular ones will be demonstrated in Barchart and word clouds.
- Sentiment Analysis
- Ratings are classified as follows: positive, neutral, or negative feelings.
- Develop algorithms including Random Forest, Naive Bayes, and Logistic Regression, to assign sentiments to given reviews.
- We will also analyze the results through accuracy, confusion matrix, classification report, etc.
- Topic Modeling
- Compile a dictionary and build a corpus from the cleaned-up reviews.
- Pursue LDA in an attempt to unearth underlying topics and their corresponding verbiage.
- Use the pyLDAvis library to surf the topics interestingly.
- Clustering
- In this regard, the text will be translated into its numerical representation using TF-IDF vectors.
- Churn out K-Means clusters for the sake of analysis of the textual data present in the reviews.
- Performed PCA to facilitate better visualization and interpretation of the data.
- Visualization
- We make use of word clouds for large clusters to bring out the most frequently mentioned terms.
- Plot clusters and topics for easy understanding of patterns and trends.
Methodology
- Collect the customer reviews and clean the data by removing any unwanted symbols, tokenizing the text, and lemmatizing the words.
- Map ratings to sentiment labels: to be categorized as either Positive, Neutral, or negative.
- Continue to train machine learning models associated with Random Forest and Naive Bayes to analyze sentiments.
- Use LDA to extract latent topics and keywords existing in customers’ comments.
- Depending on semantic patterns, K-Means clustering is to be used to group similar reviews.
- Present the result in the form of a word cloud, heat map, and some clustering plot to get a better view.
Data Collection and Preparation
Data Collection:
In this project, we collected the dataset from a public repository. If you are looking to work on a real-world problem, you can get these kinds of datasets from publicly available repositories such as Kaggle, UCI Machine Learning Repository, or company-specific data. We will provide the dataset in this project so that you can work on the same dataset.
Data Preparation Workflow:
- Import the dataset with customer reviews along with the ratings provided.
- Transform the text to lowercase and eliminate numerical information, special symbols, and punctuation marks.
- Fragment the reviews into respective words with the help of NLTK libraries.
- Omit stopwords such as ‘the’, ‘and’, and ‘is’ with the help of a built-in NLTK stopword list.
- Reduce words to their base form using WordNetLemmatizer.
- Eliminate any words that are less than three characters to reduce noise.
- Employ methods to preserve the text for rural and urban areas for later evaluation.
Code Explanation
STEP 1:
Mounting Google Drive
First, mount Google Drive to access the dataset that is stored in the cloud.
from google.colab import drive
drive.mount('/content/drive')
Installing Necessary Libraries
This code installs libraries for data processing, visualization, topic modeling, and machine learning tasks.
!pip install nltk
!pip install numpy
!pip install pandas
!pip install gensim
!pip install seaborn
!pip install xgboost
!pip install pyLDAvis
!pip install wordcloud
!pip install matplotlib
!pip install scikit-learn
Suppressing Warnings
This code disables all types of warnings to keep the output clean and focused.
# Ignore all warnings
import warnings
warnings.filterwarnings('ignore')
warnings.filterwarnings(action='ignore')
warnings.filterwarnings("ignore ", category=FutureWarning)
warnings.filterwarnings("ignore", category=DeprecationWarning)
warnings.filterwarnings("default", category=DeprecationWarning)
NLTK Data Installation
The following code ensures the availability of basic NLTK data and tools used for text splitting, lemmatization, opinion mining, and the filtration of common words.
import nltk
nltk.download('punkt')
nltk.download('omw-1.4')
nltk.download('wordnet')
nltk.download('stopwords')
nltk.download('vader_lexicon')
Importing Libraries for Text Processing, Visualization, and Machine Learning
This code is importing tools for NLP and Clustering and Classification and Dimensionality Reduction and Sentiment Analysis and Evaluation of the Performance among others.