Optimizing Chunk Sizes for Efficient and Accurate Document Retrieval Using HyDE Evaluation
Optimize document retrieval with GPT-4, using vector indexing and chunk size tuning for fast, accurate real-time and real-world AI search insights.
$4 USD
$3.00 USD
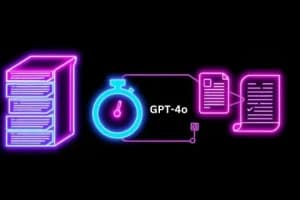
Project Outcomes
-
Demonstrates improved retrieval speed and accuracy with optimized chunk sizes.
-
Enables efficient document segmentation for faster processing.
-
Achieves high faithfulness in responses using GPT-4 evaluations.
-
Enhances relevancy assessment with tailored prompt templates.
-
Implements scalable vector indexing for effective data retrieval.
-
Automates the generation of evaluation questions from document datasets.
-
Provides detailed metrics on response time, faithfulness and relevancy.
-
Reduces overall processing time for quicker insights.
-
Facilitates real-world applications in search engines, digital libraries and customer support systems.
-
Establishes a robust framework for future generative AI retrieval projects.
You might also like
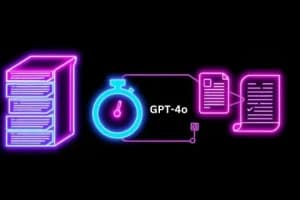
Optimizing Chunk Sizes for Efficient and Accurate Document Retrieval Using HyDE Evaluation
This project demonstrates the integration of generative AI techniques with efficient document retrieval by leveraging GPT-4 and vector indexing. It...
-with-dynamic-adjustment.webp&w=3840&q=75)
Corrective Retrieval-Augmented Generation (RAG) with Dynamic Adjustments
In the rapidly evolving field of artificial intelligence, the ability to retrieve accurate information and generate informed responses is paramount,...
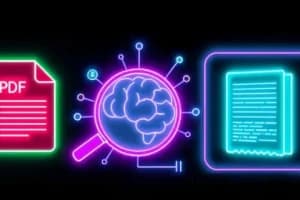
Enhancing Document Retrieval with Contextual Overlapping Windows
This project demonstrates a method to enhance document retrieval using contextually overlapping windows in a vector database. Adding surrounding context...
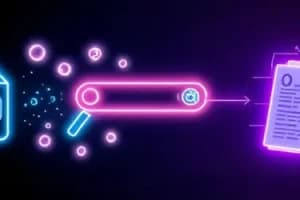
Document Augmentation through Question Generation for Enhanced Retrieval
This project focuses on document retrieval enhancement through text augmentation via question generation. The method aims to improve document search...