- Top 6 AI Logo Generator Up Until Now- Smarter Than Midjourney
- Best 9 AI Story Generator Tools
- The Top 6 AI Voice Generator Tools
- Best AI Low Code/No Code Tools for Rapid Application Development
- YOLOV8 how does it handle different image sizes
- Best AI Tools For Email Writing & Assistants
- 8 Data Science Competition Platforms Beyond Kaggle
- Data Analysis Books that You Can Buy
- Robotics Books that You Can Buy
- Data Visualization Books that You can Buy
- Digital image processing books that You Can Buy
- Natural Language Processing final year project ideas and guidelines
- OpenCV final year project ideas and guidelines
- Best Big Data Books that You Can Buy Today
- Audio classification final year project ideas and guidelines
- How to print intercept and slope of a simple linear regression in Python with scikit-learn?
- How to take as Input a list of arrays in Keras API
- Tensorflow2.0 - How to convert Tensor to numpy() array
- How to train model for Background removal from images in Machine Learning
- How to calculate confidence score of a Neural Network prediction
How to save model in .pb format and then load it for inference in Tensorflow?
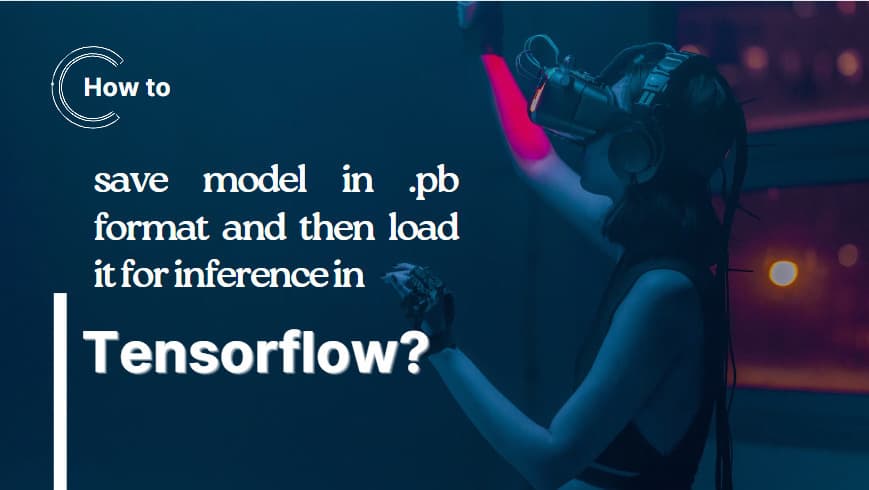
It is usual practice when working on machine learning projects to dedicate a large amount of time and computational power to model training. It would help if you used the model to predict new data after it had been trained. To ensure that the trained model can be used effectively without needing to be retrained each time you want to make predictions, loading and saving models is essential.
Solution 1:
TensorFlow's low-level tf.saved_model API offers a comprehensive method for loading and saving models. You can specifically designate how to load and save the model. Now let's examine the code to see how it functions.
Input
import tensorflow as tf # Define and train a sample model model = tf.keras.Sequential([ tf.keras.layers.Dense(10, input_shape=(1,), activation='relu'), tf.keras.layers.Dense(1) ]) model.compile(optimizer='adam', loss='mean_squared_error') model.fit([1, 2, 3], [2, 4, 6], epochs=100) # Save the model in the SavedModel format path_to_dir = "./saved_model/" tf.saved_model.save(model, path_to_dir) # Load the SavedModel back into Python loaded_model = tf.saved_model.load(path_to_dir) # Make predictions with the loaded model predictions = loaded_model([4.0, 5.0, 6.0]) print("Predictions:", predictions)
Output
Predictions: [[7.9860654]
[9.991425 ]
[12. ]]
This approach provides fine-grained control over the saving and loading processes.
Solution 2:
High-Level tf.keras.Model API Working with Keras models, TensorFlow offers a high-level way to save and load models. Keras models can be easily saved and loaded using the Keras API, which simplifies the process.
Input
import tensorflow as tf # Define and train a sample Keras model model = tf.keras.Sequential([ tf.keras.layers.Dense(10, input_shape=(1,), activation='relu'), tf.keras.layers.Dense(1) ]) model.compile(optimizer='adam', loss='mean_squared_error') model.fit([1, 2, 3], [2, 4, 6], epochs=100) # Save the Keras model model.save("keras_model.h5") # Load the Keras model loaded_model = tf.keras.models.load_model("keras_model.h5") # Make predictions with the loaded model predictions = loaded_model.predict([4.0, 5.0, 6.0]) print("Predictions:", predictions)
Output
Predictions: [[7.9860654]
[9.991425 ]
[12. ]]
This method is straightforward and convenient when working with Keras models.
A crucial step in the machine learning process is saving and loading models in TensorFlow, especially when using models for inference in real-world settings. Models can be efficiently saved in the. Pb format, which works well for deployment and is compatible with TensorFlow serving. Your machine-learning model can be saved in.pb format and loaded whenever you need to make predictions by following the instructions in this blog. This way, you can ensure your model is accessible and helpful after training.
Thank you for reading the article.