- How To Import The MNIST Dataset From Local Directory Using PyTorch
- how to split up tf.data.Dataset into x_train, y_train, x_test, y_test for keras
- How to plot confusion matrix for prefetched dataset in Tensorflow
- How to Use Class Weights with Focal Loss in PyTorch for Imbalanced dataset for MultiClass Classification
- How to solve "ValueError: y should be a 1d array, got an array of shape (3, 5) instead." for naive Bayes?
- How to create image of confusion matrix in Python
- What are the numbers in torch.transforms.normalize and how to select them?
- How to assign a name for a pytorch layer?
- How to solve dist.init_process_group from hanging or deadlocks?
- How to use sample weights with tensorflow datasets?
- How to Fine-tune HuggingFace BERT model for Text Classification
- How to Convert Yolov5 model to tensorflow.js
- Machine Learning Project: Hotel Booking Prediction [Part 2]
- Machine Learning Project: Airline Tickets Price Prediction
- Machine Learning Project: Hotel Booking Prediction [Part 1]
- Machine Learning Project Environment Setup
- Computer vision final year project ideas and guidelines
- Build Your First Machine Learning Project in Python(Step by Step Tutorial)
- Virtual assistant final year project ideas and guidelines
- Self-driving car github repositories and projects
How to train new classes on pretrained yolov4 model in darknet
Written by - Aionlinecourse1543 times views
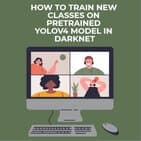
Recommended Projects
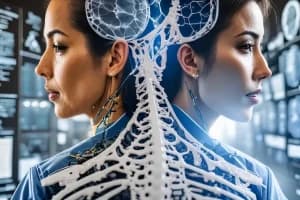
Medical Image Segmentation With UNET
Computer Vision
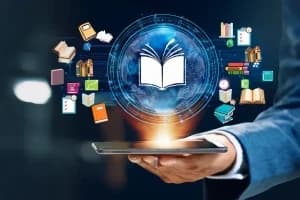
Build A Book Recommender System With TF-IDF And Clustering(Python)
Machine LearningDeep LearningNatural Language Processing
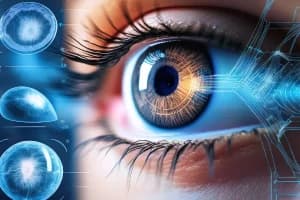
Automatic Eye Cataract Detection Using YOLOv8
Computer Vision
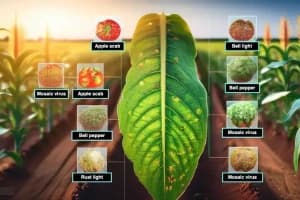
Crop Disease Detection Using YOLOv8
Computer Vision
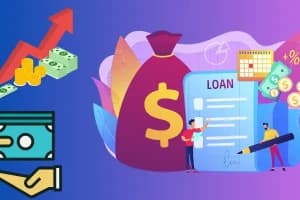
Loan Eligibility Prediction using Gradient Boosting Classifier
Machine Learning
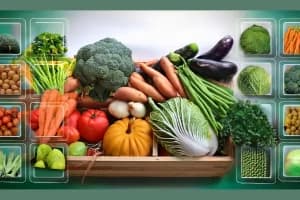
Vegetable classification with Parallel CNN model
Machine LearningDeep Learning
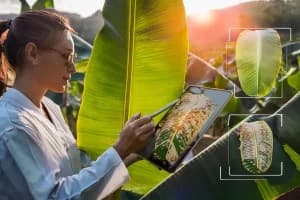
Banana Leaf Disease Detection using Vision Transformer model
Deep LearningComputer Vision