There are a few ways you can avoid reloading your machine learning (ML) model every time you call your Python script:
1. Load the model once and save it to a global variable: If you are using the same model throughout your script, you can load the model once at the beginning of your script and save it to a global variable. You can then use this global variable to make predictions without having to reload the model each time.
2. Use a persistent model: If you are using a model that can be persisted (saved to disk and loaded later), you can save the model to disk after you have trained it and then load it each time you need to make a prediction. This can be more efficient than training the model from scratch each time.
3. Use a server to host the model: If you are making many predictions and don't want to load the model each time, you can set up a server to host the model and make predictions using an API. This way, you can make predictions by sending data to the server and receiving the prediction in return, without having to load the model on the client side.
4. Use a cache: If you are making the same prediction multiple times, you can use a cache to store the results of the prediction and reuse them without having to re-run the model. This can be especially useful if your model is computationally expensive to run.
Recent Articles
- How to split data based on a column value in sklearn
- How to use sklearn ( chi-square or ANOVA) to removes redundant features
- How to graph centroids with KMeans
- How to solve ' CUDA out of memory. Tried to allocate xxx MiB' in pytorch?
- How to calculate TPR and FPR in Python without using sklearn?
- How to create a custom PreprocessingLayer in TF 2.2
- Python: How to retrive the best model from Optuna LightGBM study?
- How to predownload a transformers model
- How to reset Keras metrics?
- How to handle missing values (NaN) in categorical data when using scikit-learn OneHotEncoder?
- How to get probabilities along with classification in LogisticRegression?
- How to choose the number of units for the Dense layer in the Convoluted neural network for a Image classification problem?
- How to use pydensecrf in Python3.7?
- How to set class weights in DecisionTreeClassifier for multi-class setting
- How to Extract Data from tmdB using Python
- How to add attention layer to a Bi-LSTM
- How to include SimpleImputer before CountVectorizer in a scikit-learn Pipeline?
- How to load a keras model saved as .pb
- How to train new classes on pretrained yolov4 model in darknet
- How To Import The MNIST Dataset From Local Directory Using PyTorch
How to avoid reloading ML model every time when I call python script?
Recommended Projects
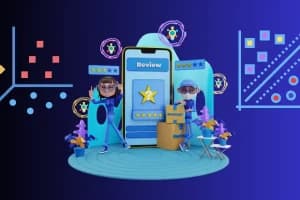
Topic modeling using K-means clustering to group customer reviews
Have you ever thought about the ways one can analyze a review to extract all the misleading or useful information?...
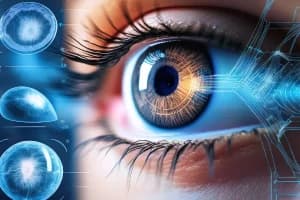
Automatic Eye Cataract Detection Using YOLOv8
Cataracts are a leading cause of vision impairment worldwide, affecting millions of people every year. Early detection and timely intervention...
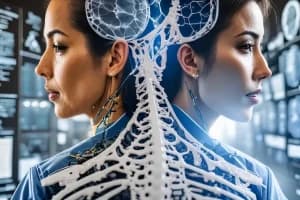
Medical Image Segmentation With UNET
Have you ever thought about how doctors are so precise in diagnosing any conditions based on medical images? Quite simply,...
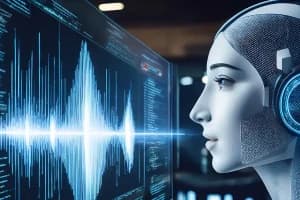
Voice Cloning Application Using RVC
Ever been curious about voice cloning? Thanks to advanced technology such as deep learning and RVC (Retrieval-based Voice Conversion), it...
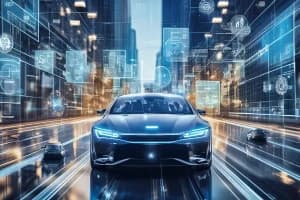
Real-Time License Plate Detection Using YOLOv8 and OCR Model
Ever wondered how those cameras catch license plates so quickly? Well, this project does just that! Using YOLOv8 for real-time...
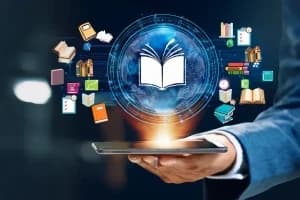
Build A Book Recommender System With TF-IDF And Clustering(Python)
Have you ever thought about the reasons behind the segregation and recommendation of books with similarities? This project is aimed...
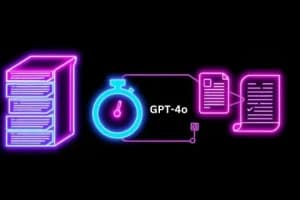
Optimizing Chunk Sizes for Efficient and Accurate Document Retrieval Using HyDE Evaluation
This project demonstrates the integration of generative AI techniques with efficient document retrieval by leveraging GPT-4 and vector indexing. It...